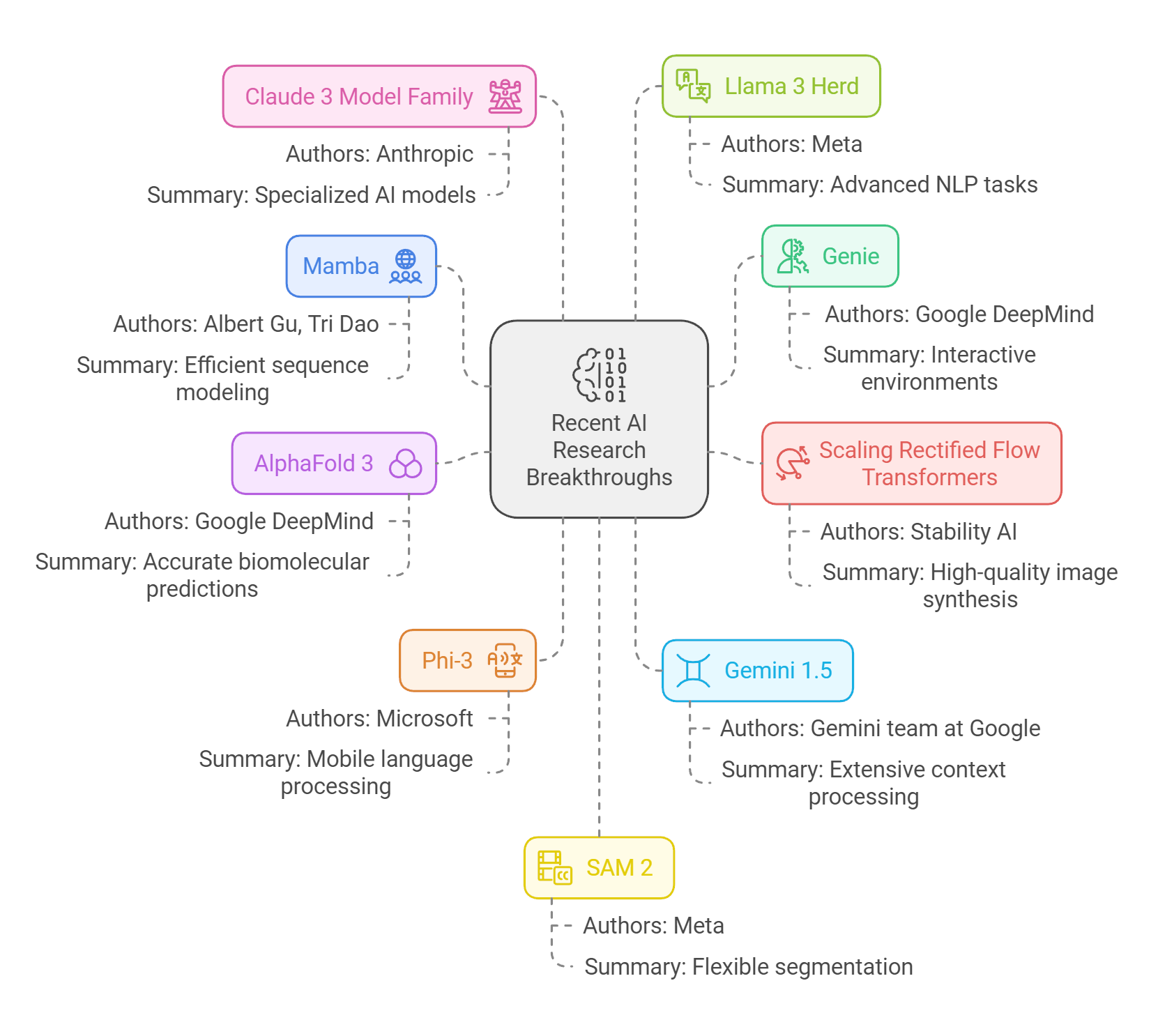
Source: https://www.topbots.com/ai-research-papers-2024/
The article “Advancing AI in 2024: Highlights from 10 Groundbreaking Research Papers” from TOPBOTS discusses ten significant AI research papers that have expanded the frontiers of artificial intelligence across various domains. These studies, produced by leading research labs such as Meta, Google DeepMind, Stability AI, Anthropic, and Microsoft, showcase innovative approaches in areas including large language models, multimodal processing, video generation and editing, and the creation of interactive environments.
1. Mamba: Linear-Time Sequence Modeling with Selective State Spaces
- Authors: Albert Gu (Carnegie Mellon University) and Tri Dao (Princeton University)
- Summary: Mamba introduces a neural architecture for sequence modeling that addresses the computational inefficiencies of Transformers while matching or exceeding their modeling capabilities. It features a novel selection mechanism within state space models, enabling the filtering of irrelevant information and the indefinite retention of critical context. This design allows for true linear scaling in sequence length and up to three times faster computation on modern GPUs compared to prior state space models.
2. Genie: Generative Interactive Environments
- Authors: Google DeepMind
- Summary: Genie presents a framework for creating interactive environments using generative models. This approach facilitates the development of dynamic and responsive virtual settings, enhancing the interaction between AI systems and their environments.
3. Scaling Rectified Flow Transformers for High-Resolution Image Synthesis
- Authors: Stability AI
- Summary: This research focuses on scaling Rectified Flow Transformers to improve high-resolution image synthesis. The advancements lead to the generation of high-quality images, pushing the boundaries of what is achievable in image synthesis.
4. Accurate Structure Prediction of Biomolecular Interactions with AlphaFold 3
- Authors: Google DeepMind
- Summary: AlphaFold 3 builds upon its predecessors to enhance the accuracy of predicting biomolecular interactions. This development holds significant implications for fields such as drug discovery and molecular biology.
5. Phi-3 Technical Report: A Highly Capable Language Model Locally on Your Phone
- Authors: Microsoft
- Summary: Phi-3 is a language model designed to operate efficiently on mobile devices. It brings advanced language processing capabilities to smartphones, enabling sophisticated AI applications without relying on cloud-based resources.
6. Gemini 1.5: Unlocking Multimodal Understanding Across Millions of Tokens of Context
- Authors: Gemini team at Google
- Summary: Gemini 1.5 enhances multimodal understanding by processing extensive contexts across various modalities. This capability improves the model’s performance in tasks that require integrating information from multiple sources.
7. The Claude 3 Model Family: Opus, Sonnet, Haiku
- Authors: Anthropic
- Summary: The Claude 3 series comprises models tailored for different applications, each optimized for specific tasks. This specialization allows for more efficient and effective AI solutions across diverse use cases.
8. The Llama 3 Herd of Models
- Authors: Meta
- Summary: Llama 3 represents a suite of models that advance the state of large language models. These models offer improved performance and versatility in natural language processing tasks.
9. SAM 2: Segment Anything in Images and Videos
- Authors: Meta
- Summary: SAM 2 introduces a model capable of segmenting any object within images and videos, enhancing computer vision applications by providing more accurate and flexible segmentation capabilities.
10. Movie Gen: A Cast of Media Foundation Models
- Authors: Meta
- Summary: Movie Gen encompasses a collection of media foundation models designed to generate and edit video content. This suite of tools facilitates the creation of high-quality media, advancing the field of AI-generated content.
These papers collectively represent significant strides in AI research, offering innovative solutions and expanding the potential applications of artificial intelligence across various sectors.