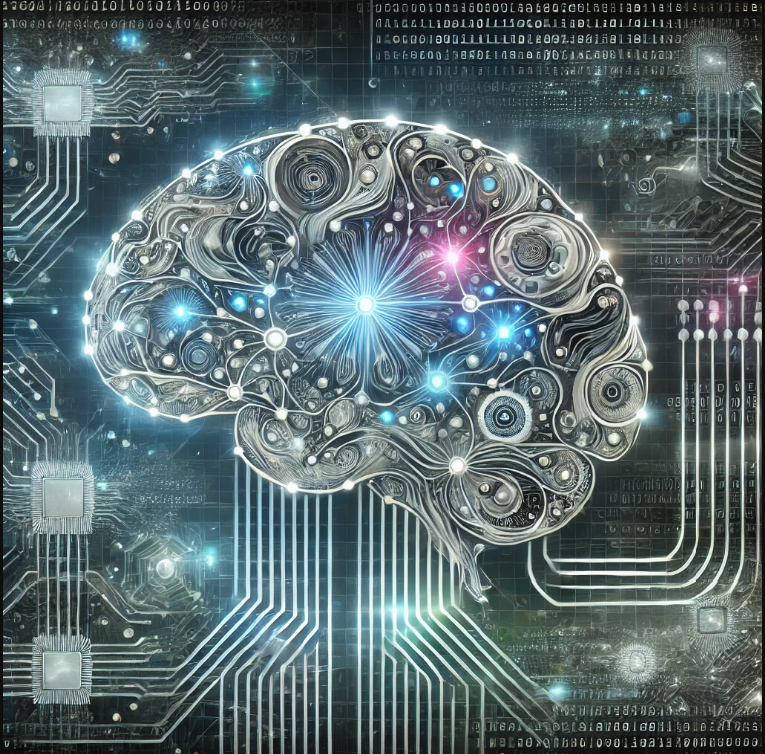
Neuromorphic computing, inspired by the structure and functionality of the human brain, has shown promise in energy efficiency and parallel processing. However, it has yet to make a significant impact in the mainstream AI development currently dominated by large-scale models and data centers. This article examines the current status and future potential of neuromorphic computing amidst evolving AI trends, such as lightweight AI models like DeepSeek.
Current Status and Challenges of Neuromorphic Computing
Neuromorphic computing aims to replicate the brain’s efficiency in processing information, offering advantages in power consumption and real-time processing. However, several challenges hinder its adoption in mainstream AI:
- Lack of Generalization: Neuromorphic systems excel in specific applications, such as processing sensor data with spiking neural networks, but they struggle to match the versatility of general-purpose AI models like OpenAI’s o1.
- Immature Development Ecosystem: The tools and frameworks for developing neuromorphic hardware and software are still in their infancy, limiting their competitiveness with GPUs and TPUs optimized for deep learning.
- Ecosystem Barriers: The current AI ecosystem is heavily reliant on cloud computing and large-scale data infrastructure. Integrating neuromorphic computing into this framework requires significant changes.
The Rise of Lightweight AI Models Like DeepSeek
The emergence of lightweight AI models like DeepSeek, which rivals the performance of larger models with minimal computational resources, has raised questions about the future direction of AI development. DeepSeek highlights key considerations:
- Efficiency in Computing Resources: Neuromorphic computing’s strengths in low-power, efficient processing align well with the success of lightweight AI models like DeepSeek.
- Potential for Edge Devices: Neuromorphic systems are well-suited for edge applications, where power efficiency and local processing are critical.
The Role of Neuromorphic Computing Amid U.S. Investments in AI
The United States has made significant investments in AI development, such as the “Stargate Project,” which includes the use of nuclear power to sustain large-scale data centers. Neuromorphic computing currently occupies a niche role in this landscape:
- Short-Term Impact: Neuromorphic computing has yet to be integrated into the infrastructure supporting large-scale AI systems, as its capabilities are not yet sufficient for the extensive parallelism and versatility required.
- Long-Term Potential: Neuromorphic computing could play a critical role in energy-efficient AI systems and specialized applications. It may eventually complement sustainable energy solutions, such as nuclear power, to optimize energy use.
The Potential Role of Neuromorphic Computing in Future AI
As the feasibility of large-scale AI systems comes into question, neuromorphic computing could address several challenges:
- Decentralized AI Systems: Neuromorphic technology can thrive in edge computing and IoT devices, reducing dependence on centralized data centers.
- Energy Efficiency: With AI systems requiring ever-growing power, the low-power characteristics of neuromorphic computing could offer significant advantages.
- Exploration of New AI Architectures: Neuromorphic systems may enable the development of novel algorithms and models that go beyond traditional deep learning.
Conclusion
Neuromorphic computing is not yet a major player in the mainstream AI landscape dominated by large-scale models and centralized data centers. However, its potential in energy-efficient and specialized applications positions it as a technology to watch. The success of lightweight AI models like DeepSeek suggests a growing demand for “smaller, smarter AI,” which could open the door for neuromorphic computing to influence the future of AI development.
While it may take time for neuromorphic systems to achieve the versatility and scale of current AI models, their long-term potential could lead to breakthroughs in how AI is developed and deployed.