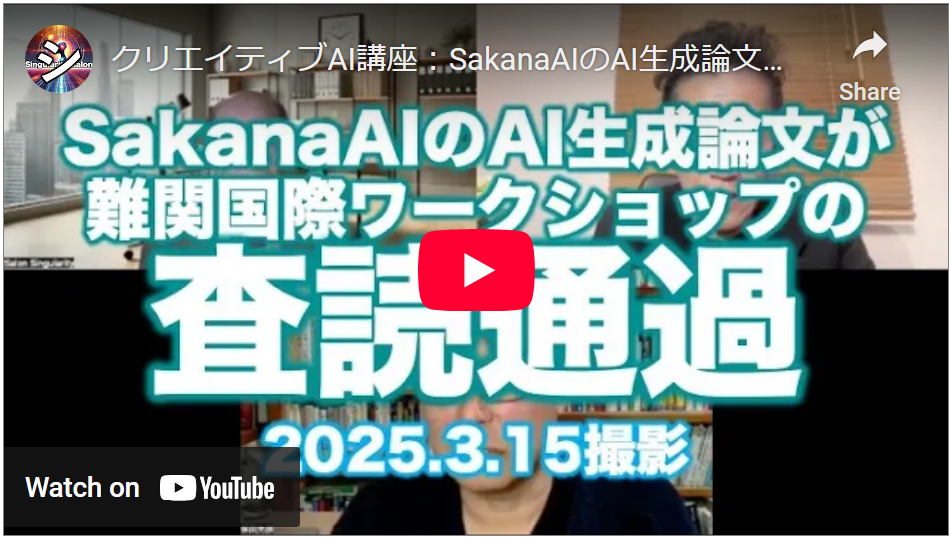
In a landmark event for the scientific community, Sakana AI’s AI-generated research paper has successfully passed peer review at an international conference. This achievement marks a significant milestone in the intersection of artificial intelligence and academic research, paving the way for future innovations in the field.
Introduction to Sakana AI’s Achievement
Sakana AI has achieved a remarkable breakthrough in the realm of artificial intelligence and academic research. The AI Scientist V2 has successfully generated a research paper that has passed peer review at a prestigious international conference. This accomplishment signifies not just a technological advancement, but a pivotal moment in how we perceive the capabilities of AI in contributing to scholarly work.
Significance of the International Conference
The acceptance of Sakana AI’s paper at the International Conference on Learning Representations (ICLR) is monumental. ICLR is one of the top conferences in the field of machine learning and artificial intelligence, alongside others like NeurIPS and ICML. This recognition highlights the increasing integration of AI in academic settings and its potential to reshape the landscape of research.
The Role of AI Scientist V2
The AI Scientist V2 played a crucial role in generating the research paper. This advanced version of Sakana AI’s system is designed to formulate scientific hypotheses, conduct experiments, and analyze data—all autonomously. The evolution of the AI Scientist represents a significant leap in machine learning capabilities, showcasing how AI can now contribute meaningfully to scientific inquiry without human intervention.
Submission Process and Peer Review
The process of submitting the paper was meticulously planned. Out of 43 generated papers, Sakana AI selected the top three for submission to ICLR. These submissions underwent a rigorous peer review process, where experts evaluated their quality without knowing which papers were AI-generated. This approach not only tested the AI’s capabilities but also adhered to the standards of academic integrity.
The Unprecedented Nature of AI-Generated Papers
What sets this achievement apart is that the paper was entirely generated by AI, without any human edits or modifications. While AI-assisted papers are not new, this was a fully autonomous creation. The AI Scientist V2 formulated hypotheses, designed experiments, and crafted the manuscript, demonstrating that AI can handle complex scientific tasks traditionally reserved for human researchers.
Results of the Submission
From the three submitted papers, one was accepted after passing the peer review process, while the other two did not meet the acceptance criteria. Nonetheless, the fact that any AI-generated paper was accepted is a significant milestone, indicating that the quality of the research met or exceeded the standards set by human authors in the field.
The Title and Content of the Accepted Paper
The accepted paper, titled “Computer Reguralization: Unone Expected Obstacles in Enhancing Neural Network Generalization,” explores new methods for improving the generalization capabilities of neural networks. This groundbreaking study examines the unexpected challenges encountered when applying novel regularization techniques in neural network training. Although the paper is not publicly available, its insights contribute to the ongoing discourse on AI’s role in advancing machine learning methodologies.
The Importance of the Peer Review Process
The peer review process is fundamental to maintaining the integrity and quality of scientific research. It serves as a gatekeeper, ensuring that only research meeting rigorous standards is published. For AI-generated papers, this process becomes even more crucial.
By passing through peer review, Sakana AI’s paper demonstrates that AI can not only generate research but can also meet the expectations set by human researchers. This acceptance underscores the importance of having a robust evaluation framework to assess AI contributions to science.
Peer review fosters credibility. When experts in the field evaluate the work, it provides assurance to the scientific community that the findings are sound and reliable. This is vital for AI-generated research, as it must establish trust to be taken seriously.
Evaluating AI Contributions
As AI continues to evolve, it is essential to develop specific criteria for evaluating AI-generated research. Traditional metrics may not fully capture the nuances of AI’s contributions. Therefore, a tailored approach to peer review is necessary to address the unique challenges posed by AI in research.
- Transparency: Reviewers must understand how the AI generated the research and the methodologies used.
- Reproducibility: Findings should be replicable, which is a cornerstone of scientific research.
- Innovation: The research should contribute new knowledge or methods to the field.
Challenges Faced by AI in Research
While the acceptance of AI-generated papers is a significant step forward, challenges remain. The technology is still in its infancy, and there are hurdles that need to be addressed for AI to become a mainstay in scientific research.
One of the primary challenges is the potential for errors in AI-generated content. For instance, mistakes in citations or factual inaccuracies can undermine the credibility of the research. As seen in Sakana AI’s paper, even minor errors can lead to questions about the reliability of the findings.
Another challenge is the inherent bias in AI systems. AI learns from existing data, which can perpetuate biases present in the training data. This could lead to skewed results or reinforce existing stereotypes within scientific literature.
Mitigating Challenges
To combat these challenges, ongoing monitoring and refinement of AI systems are crucial. Continuous training with diverse datasets can help minimize bias, while regular audits can identify and rectify errors in generated content.
- Training Diversity: Incorporate a wide range of sources to reduce bias.
- Quality Control: Implement rigorous checks to catch errors before submission.
- Collaborative Efforts: Foster partnerships between AI developers and researchers to align goals and standards.
Future Implications for AI in Science
The future of AI in scientific research is promising, with the potential to revolutionize how research is conducted and disseminated. As AI technology advances, we can expect more sophisticated algorithms capable of tackling complex scientific questions.
AI could streamline the research process, from hypothesis generation to data analysis, freeing researchers to focus on creative and critical thinking. This shift could lead to faster discoveries and innovations across various scientific disciplines.
Moreover, the integration of AI in research can enhance collaboration among scientists worldwide. AI systems can facilitate communication and data sharing, breaking down traditional barriers in research collaboration.
Expanding Research Horizons
As AI continues to develop, it will open new avenues for exploration. Researchers might utilize AI to identify patterns in data that humans may overlook, leading to groundbreaking discoveries.
- Interdisciplinary Research: AI can bridge gaps between fields, encouraging collaboration across disciplines.
- Real-Time Analysis: With AI, researchers can analyze data in real time, leading to quicker conclusions and adaptations.
- Personalized Research: AI can tailor research approaches to individual needs or specific populations, enhancing relevance and impact.
Sakana AI’s Vision for the Future
Sakana AI envisions a future where AI is an integral part of the scientific community. The organization believes that AI can significantly enhance the quality of research by providing unique insights and efficiencies.
By continuously improving its AI systems, Sakana AI aims to push the boundaries of what is possible in research. The goal is to create a collaborative environment where AI and human researchers work together to solve the world’s most pressing challenges.
Moreover, Sakana AI is committed to maintaining ethical standards in AI research. This involves being transparent about the capabilities and limitations of AI while ensuring that the research generated is of the highest quality.
Commitment to Ethical AI
As AI becomes more prevalent in research, ethical considerations will be paramount. Sakana AI is dedicated to ensuring that its AI systems are used responsibly and that the benefits of AI research are shared broadly.
- Ethical Guidelines: Establish and adhere to guidelines that promote responsible AI use.
- Community Engagement: Involve stakeholders in discussions about the ethical implications of AI in research.
- Education and Training: Provide resources to educate researchers about the responsible use of AI.
The Need for Transparency in AI Research
Transparency is essential in AI research to build trust within the scientific community and the public. Researchers must disclose how AI systems operate, the data used for training, and the methodologies employed in generating research outputs.
By being transparent, researchers can foster accountability and allow others to replicate findings. This is crucial for verifying the validity of AI-generated research and enhancing its credibility.
Furthermore, transparency can help mitigate biases inherent in AI systems. When researchers openly share their processes and data, it allows for scrutiny and improvement, ensuring that AI contributes positively to scientific advancement.
Building a Framework for Transparency
Creating a culture of transparency in AI research requires collective effort. Researchers, institutions, and AI developers must work together to establish best practices and standards.
- Open Access Publishing: Encourage the publication of AI-generated research in open-access formats to increase accessibility.
- Data Sharing: Promote the sharing of datasets used in AI training to facilitate understanding and replication.
- Documentation: Maintain thorough documentation of AI processes to enable scrutiny and improvement.
Potential for AI to Transform Scientific Publishing
The landscape of scientific publishing is on the brink of transformation, thanks to AI. With AI systems capable of generating high-quality research, the traditional publishing model may need to adapt to accommodate these advancements.
AI can streamline the submission and review process, making it more efficient. Automated systems can assist in initial screenings and even facilitate peer review by matching papers with appropriate reviewers.
Moreover, AI can help identify trends in research, guiding funding and publishing decisions. This could lead to a more dynamic and responsive publishing environment that better serves the scientific community’s needs.
Reimagining the Publishing Process
As AI continues to influence scientific publishing, we must rethink how we approach the dissemination of knowledge. Embracing AI’s potential can lead to a more inclusive and accessible research landscape.
- Adaptive Publishing Models: Develop publishing models that integrate AI-generated content while maintaining quality standards.
- Collaborative Platforms: Create platforms that facilitate collaboration between AI and human researchers in the publishing process.
- Continuous Learning: Implement systems that learn from feedback to improve the efficiency and quality of the publishing process.
Conclusion and Call for Open Research
The acceptance of AI-generated research marks a pivotal moment in the scientific community. As we move forward, it is essential to embrace the potential of AI while addressing the challenges it presents.
Transparency, ethical considerations, and collaboration will be crucial in shaping the future of AI in research. By fostering an environment where AI and human researchers can work together, we can unlock new possibilities for scientific advancement.
Let us advocate for open research and the responsible use of AI in science. Together, we can harness the power of AI to drive innovation and address the world’s most pressing challenges.