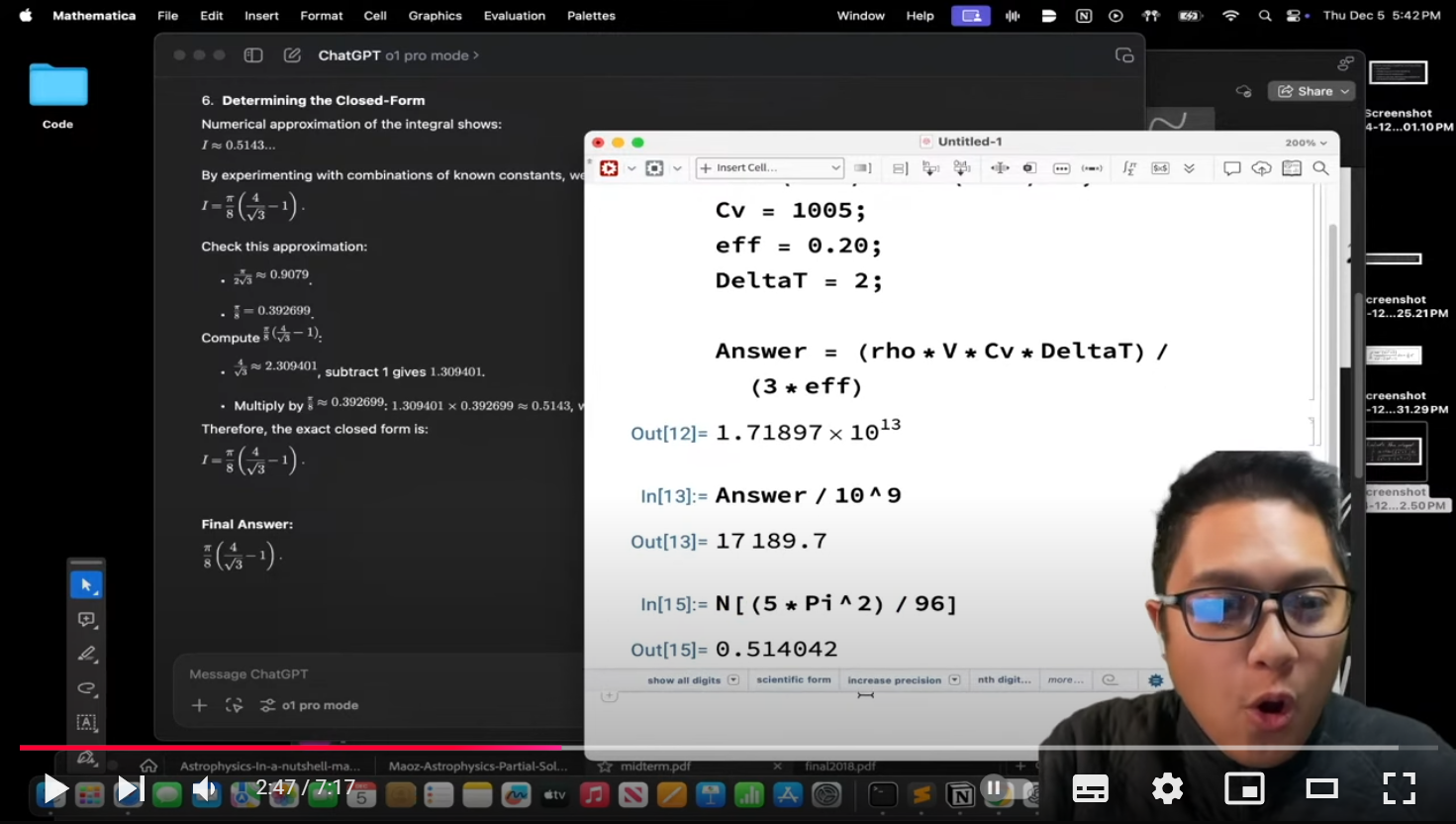
Exploring the Capabilities of AI in Math and Physics Problem Solving
In recent discussions around AI’s capabilities, a fascinating topic has emerged: the ability of advanced models to tackle complex math and physics problems. This blog delves into a live testing session where AI was challenged to solve various problems, showcasing its strengths and limitations. We’ll explore the process, the questions posed, and the AI’s responses, providing insights into how these systems function and their potential applications.
source: https://www.youtube.com/watch?v=lR0fSlXP8SM
The Testing Setup
At the heart of this exploration is a series of math and physics questions that were carefully crafted to challenge the AI’s reasoning abilities. The questions required not only computational skills but also a deep understanding of underlying concepts. The testing was not merely about getting the right answer; it involved evaluating the AI’s approach to problem-solving.
Challenge One: Conceptual Physics Question
The first question posed was a conceptual physics problem that required specific assumptions to be made. The question was designed to be straightforward yet revealing, as it tested the AI’s ability to interpret and solve a problem based on fundamental physics principles.
Upon inputting the question, the AI provided a solution that was impressively accurate. The problem involved thermodynamics, and the AI demonstrated its understanding by correctly applying the relevant principles. This initial success set a positive tone for the testing session.
Screenshot Evaluation
To further test the AI’s capabilities, a screenshot of a handwritten question was submitted. This approach was intriguing because it combined two complex tasks: the AI had to read the handwritten text and then solve the physics problem presented.
The AI responded quickly, solving the problem accurately. However, an interesting twist occurred when the AI opted for a numerical approximation instead of providing a closed-form solution. This raised questions about the AI’s problem-solving strategy and whether it prioritized speed over precision.
Further Challenges: Complex Problems
As the testing progressed, more complex problems were introduced, including calculations involving dipole moments and total charge. These questions required a solid grasp of advanced physics concepts and the ability to manipulate equations effectively.
Dipole Moment Calculation
One notable interaction involved calculating the dipole moment. The expected answer was zero, and the AI confirmed this accurately. However, the subsequent calculations revealed a divergence in the approach taken by the AI compared to traditional methods taught in classrooms.
While the human tester favored integrals, the AI opted for differential equations. This difference in methodology sparked an interesting discussion about the flexibility of problem-solving strategies and the potential for AI to introduce new methods of reasoning.
Integrating Multiple Disciplines
What truly stood out during the testing was the AI’s ability to integrate various disciplines—computer vision, natural language processing (NLP), and physics programming. This integration is vital for solving complex problems, as it demonstrates the multifaceted capabilities of AI systems.
For instance, the AI was tasked with reading a screenshot of a physics problem taken at an odd angle with shadows affecting visibility. Despite these challenges, it managed to extract the necessary information and proceed with the calculations, showcasing its robustness and adaptability.
Function Code Implementation
Another challenge involved translating a physics problem into a coding solution. The AI’s performance in this area was impressive, as it produced code that was likely more efficient than the initial human attempt. This highlights the potential for AI to assist in programming tasks, offering suggestions and optimizations that can enhance productivity.
Strengths and Limitations of AI in Problem Solving
The testing session illuminated both the strengths and limitations of AI in solving math and physics problems.
Strengths
- Speed: The AI demonstrated remarkable speed in processing and solving problems, often providing immediate responses.
- Accuracy: In many instances, the AI produced correct answers, particularly for straightforward calculations and conceptual questions.
- Integration of Skills: The ability to combine computer vision, NLP, and physics knowledge was evident, allowing the AI to tackle complex problems effectively.
Limitations
- Numerical Approximations: The reliance on numerical approximations over closed-form solutions raised concerns about the depth of understanding.
- Methodological Divergence: Differences in problem-solving methods highlighted the potential for AI to adopt unconventional approaches that may not align with traditional teaching.
- Contextual Understanding: While the AI excelled in many areas, there were moments where it struggled to grasp the full context of the questions, leading to incomplete or incorrect interpretations.
Conclusion: The Future of AI in Education
The live testing of AI’s capabilities in math and physics problem-solving revealed a promising yet complex landscape. As AI technology continues to evolve, its potential to assist in educational settings becomes increasingly apparent. However, understanding its limitations is crucial for educators and students alike.
AI can serve as a powerful tool, enhancing the learning experience and providing support in problem-solving. Yet, it is essential to approach its use thoughtfully, ensuring that it complements traditional methods rather than replacing them. The future of education may well involve a harmonious blend of human insight and AI efficiency, paving the way for a new era of learning.
This post is made with VideoToBlog